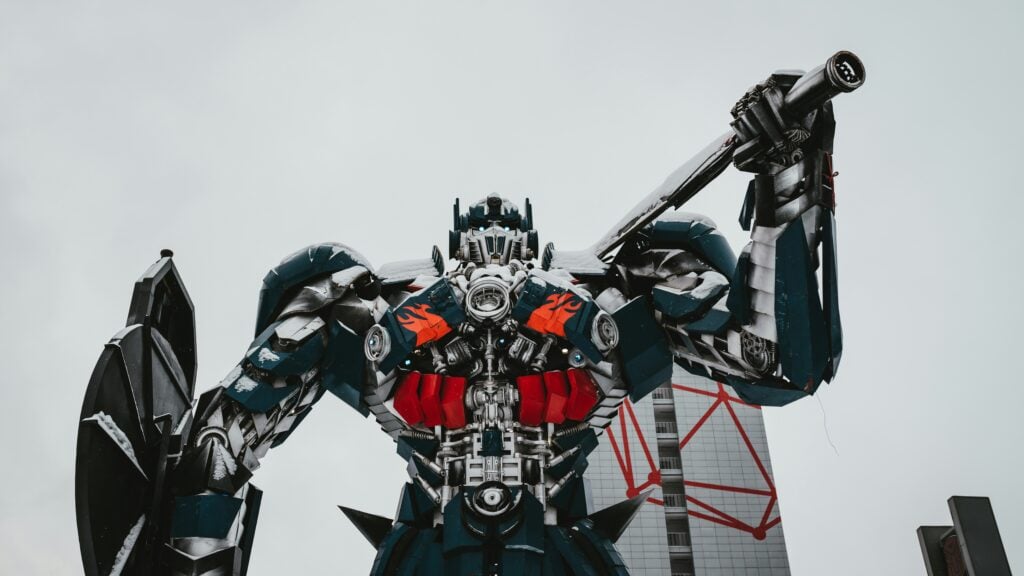
จากกระแสการมาของ AI ตัวเก่งที่ทำให้หลายๆสายงานใช้ชีวิตได้ง่ายขึ้นและยากขึ้นตามๆกันไปคือการมาของ ChatGPT จากทีม OpenAI ที่นักเรียนสามารถสั่ง ChatGPT ให้เขียนเรียงความไปส่งอาจารย์ได้โดยไม่ได้ลอกใครมา หรือจะเป็นการเขียนโค้ดส่งการบ้านของเด็กมหาลัยฯ หรือจะเป็นการสอบถามสูตรอาหารพร้อมขั้นตอนการปรุงอาหาร ที่เป็นแรงกระเพื่อมไปหลายๆวงการ ทางผู้เขียนเองก็ตะลึงในความสามารถของ ChatGPT เช่นกัน แต่ทางผู้เขียนจะขอพูดถึงการทำงานของ ChatGPT ในโอกาสหน้า ในครั้งนี้เราจะมาพูดถึงพัฒนาการของโมเดลทางภาษา (Language Models : LM) ในด้าน Natural Language Processing : NLP ที่ทำให้เกิด ChatBOT ในรูปแบบต่างๆมากมาย เช่น Alexa, Siri, Sparrow, รวมถึง ChatGPT ซึ่งมีทั้งความเก่ง ความฉลาด และความแม่นยำ
ในปี 2013 (10 ปีก่อนปัจจุบัน) เป็นปีของ Word2Vec เหล่านักวิจัยด้าน NLP ให้ Convolutional Neural Network Model: CNN เรียนรู้เพื่อหาความคล้ายคลึงหรือความเหมือนของคำแต่ละคำตามการกระจายตัวของแต่ละคำในคลังข้อมูลภาษา โดยการเปลี่ยนคำให้กลายเป็นเวกเตอร์ ซึ่งคำแต่ละคำที่อยู่ใกล้กัน คือคำที่มีความหมายใกล้เคียงกัน มีบริบทเดียวกัน หรือมีความคล้ายคลึงกัน ดังตัวอย่างที่ปรากฏในรูป 1
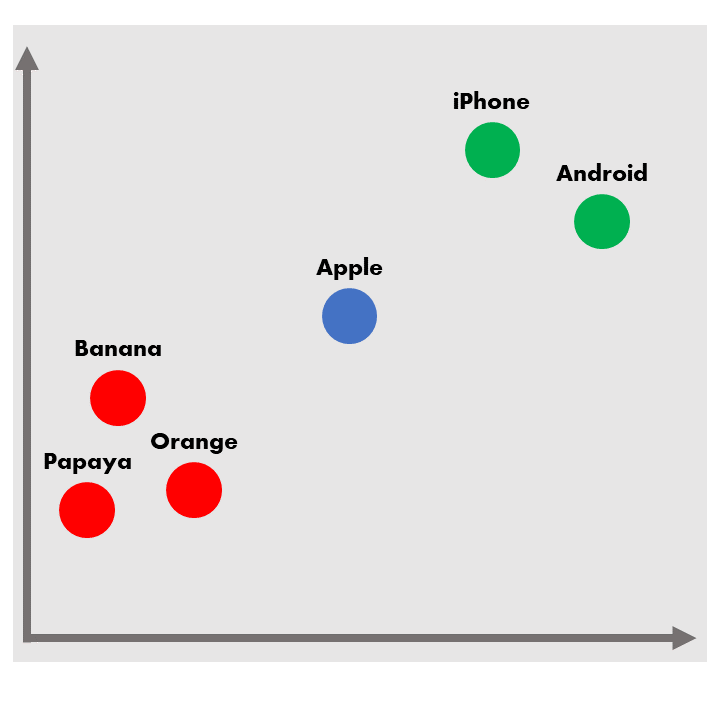
ถ้าสมมติว่าเราเปลี่ยนคำว่า “Apple” ให้กลายเป็นเวกเตอร์แล้ววาดลงบนพื้นที่ 2 มิติ Word2Vec จะบอกว่าคำที่คล้ายคลึง หรือพบเจอได้บ่อยเมื่อมีคำว่า “Apple” คือ “iPhone” และ “Android” จะเห็นได้ว่าทั้งสองคำอยู่ในบริบทของมือถือ และคำว่า “Apple” กลายเป็นชื่อแบรนด์สินค้า ในขณะเดียวกันตัว Word2Vec เองก็แสดงคำที่ลักษณะใกล้เคียงกันเช่น “Banana” “Orange” และ “Papaya” ให้อยู่ใกล้กับ “Apple” เช่นเดียวกันแต่เป็นบริบทของผลไม้ จะเห็นได้ว่า Word2Vec นั้นยังแบ่งคำว่า “Apple” ในบางเนื้อหาให้ชัดเจนไม่ได้ดีนัก หากนำไปใช้ใน Classification Model อาจทำให้เกิดความคลาดเคลื่อนได้ค่อนข้างสูง จึงทำให้ไปสู่การพัฒนาขั้นต่อไปของการใช้ Neural Network
ในปี 2014-2015 เป็นปีที่มีการนำมาใช้ของ Recurrent Neural Network : RNN เนื่องจากตัวโมเดลนั้นสามารถแก้ปัญหา Classification ได้ดี แล้วยังสามารถแก้ปัญหา Sequence-2-Sequence (Seq2Seq) ได้ดีอีกด้วย ซึ่งโจทย์ปัญหาที่พบเจอได้บ่อยๆในด้าน NLP ของ Seq2Seq คือ การแปลภาษา ยกตัวอย่างการแปลภาษาจากภาษาอังกฤษมาเป็นภาษาไทย ดังประโยคในตัวอย่าง “He is a student.” ตัว Encoder นั้นจะทำการเข้ารหัสตามลำดับของคำในประโยคโดยเริ่มจาก “He” ไปจนถึง “student” หลังจากนั้นก็จะสร้าง vector สำหรับประโยคนี้เพื่อนำไปถอดรหัสผ่านตัว Decoder ให้แปลงเป็นภาษาไทยว่า “เขาเป็นนักเรียน” จะเห็นได้จากตัวอย่างว่า RNN โมเดลที่ทำการแปลภาษานั้นสามารถทำได้ดี แต่ทว่าการแปลภาษาของ RNN โมเดลนั้นก็ยังไม่เป็นผลที่มีประสิทธิภาพดีเท่าไหร่นักถ้าประโยคที่เรานำไม่ได้มีแค่ 4 คำ ตัวโมเดลแปลภาษาจะมีปัญหาเกิดขึ้นสำหรับประโยคที่มีความยาวมากๆ, การแปลขนาดย่อหน้า, หรือขนาดหนังสือเป็นเล่ม ที่จะต้องไล่เข้ารหัสที่ละคำและถอดรหัสที่ละคำ
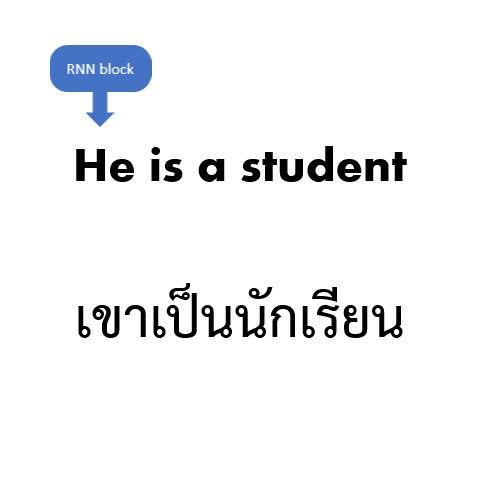
ทำให้ในช่วงปี 2015-2016 มีการเริ่มใช้กลไกการสนใจ (Attention Mechanism) คือการบอกว่าในประโยคนี้ คำนี้มีความสำคัญในการใช้แก้โจทย์ปัญหาจึงต้องสนใจคำนี้เป็นพิเศษ แทนที่จะสนใจทั้งโครงสร้างของประโยค ทางผู้เขียนขอยกตัวอย่างการใช้กลไกการสนใจเป็นรูปภาพและตัวหนังสือ เช่น “A woman is walking on the rocks” กลไกการสนใจนี้เปรียบเสมือนการโฟกัสในรูปภาพที่เรามอง คือถ้าเราให้ความสนใจกับผู้หญิงในรูปเป็นหลัก พื้นที่รอบๆก็จะหม่นลง เช่นเดียวกับตัว Attention ในประโยคข้างต้นคือ “He is a student” ตัว Self-Attention เห็นว่าความน่าสนใจของประโยคนี้คือคำว่า “student” จึงมีการนำกลไกการสนใจไปใช้ควบคู่กับ RNN ในการพัฒนาโมเดลขึ้น
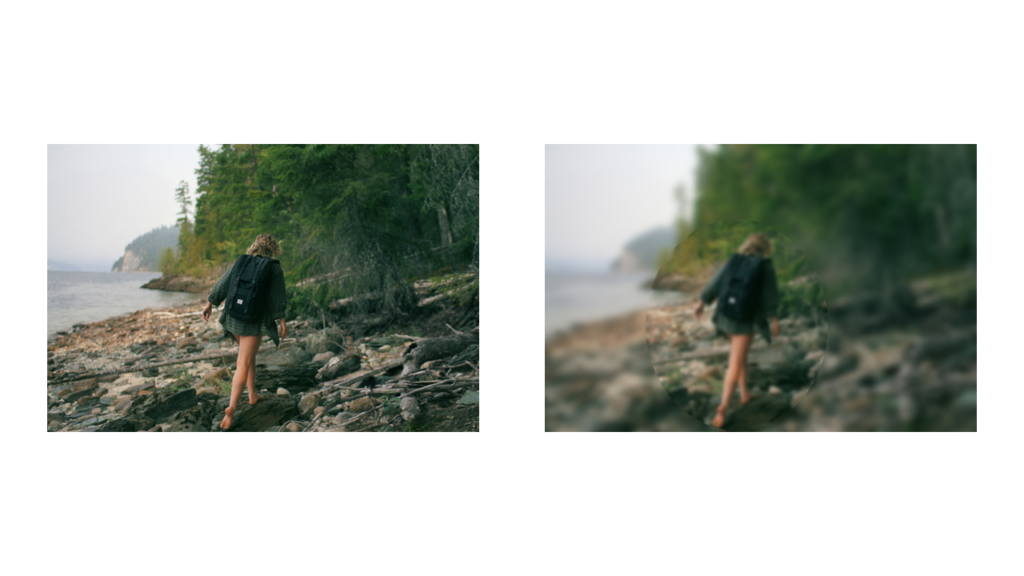
จนในที่สุดปี 2017 มีการตีพิมพ์งานวิจัยที่ชื่อว่า “Attention is All You Need” ออกมาบอกว่า เราไม่ต้องใช้ RNN block หรอก สิ่งที่เราจำเป็นจริงๆคือแค่ตัว Attention ทำให้นำไปสู่การสร้าง Neural Network ตัวใหม่ที่ชื่อว่า Transformers ที่เป็นโมเดลที่ใช้กันอย่างแพร่หลายในปัจจุบัน โดยตัวโครงสร้างของ Transformers นั้นประกอบด้วย 2 ส่วนหลักๆเช่นเดียวกับตัว RNN คือ Encoder และ Decoder แต่แตกต่างกันตรงที่ Transformers นั้นสามารถทำแบบคู่ขนานได้ (Parallelization) แทนที่จะอ่านที่ละคำตามแบบ RNN ตัว Transformers สามารถอ่านทั้งประโยคหรือทั้งย่อหน้าได้ ในส่วนของ Encoder นั้นจะเป็นการฝึกให้เข้าใจโครงสร้างทางภาษาซึ่งอาจจะประกอบไปด้วย Part of Speech (POS tagging), Semantic Roles, Coreference เป็นต้น และส่วนของ Decoder นั้นจะเป็นการสร้างคำจากประโยคที่ถูกใส่เข้ามาเพื่อตอบโจทย์ของโมเดล
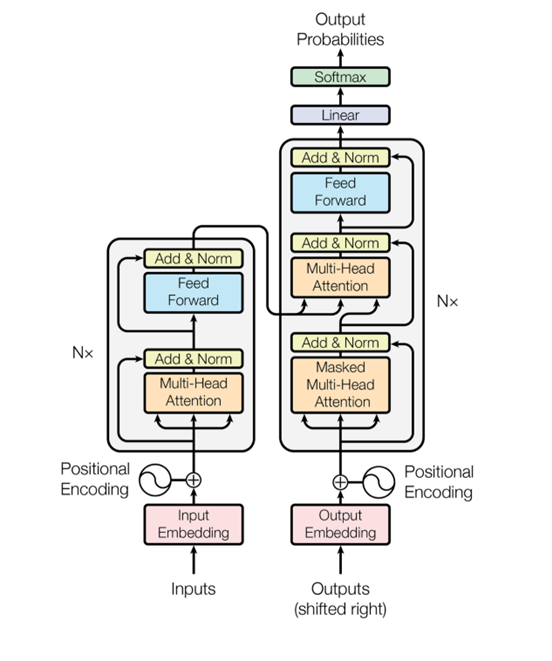
ตัว Transformers นั้นนอกจากจะใช้การเข้ารหัสตำแหน่ง (Positional Encoding) และกลไกการสนใจ (Attention Mechanism) แล้ว สิ่งที่เป็นจุดเปลี่ยนคือกลไกการสนใจตนเอง (Self-Attention Mechanism) จุดนี้ทางผู้เขียนขอย้อนกลับไปถึงตัวอย่างของคำว่า “Apple” ในตอนต้น ในรูปที่ 5 ตัว Self-Attention นั้นจะอ้างอิงความหมายของ “Apple” จากคำรอบๆตัวเอง ประโยคแรกให้ความสนใจไปที่ “fruit” และในประโยคที่สองให้ความสนใจไปที่ “cellphone” ในส่วนนี้จะช่วยให้ตัว transformers นั้นเรียนรู้โครงสร้างทางภาษาและความหมายของคำ ๆ นั้นได้มากขึ้น
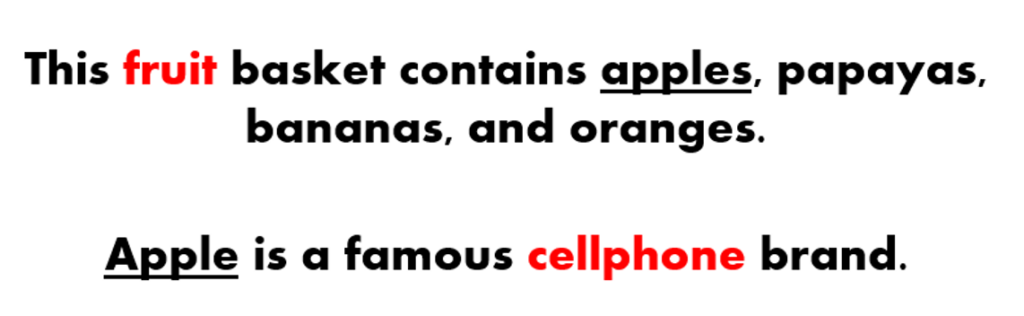
ทั้งหมดนี้เป็นเพียงแค่ที่มาของตัว Transformers ซึ่งปัจจุบันถูกพัฒนาและนำไปใช้เป็นต้นแบบและพื้นฐานในโมเดลทางด้านภาษาอย่างหลากหลาย ในโอกาสหน้าทางผู้เขียนจะมาเจาะลึกถึงตัว Transformers ว่าลำดับขั้นตอนในการดำเนินการของ Transformers เป็นอย่างไร และมีการคำนวณเบื้องหลังอย่างไร
บทความโดย อมร โชคชัยสิริภักดี
ตรวจทานและปรับปรุงโดย อนันต์วัฒน์ ทิพย์ภาวัต
ที่มา :
- What is a Transformer? An Introduction to Transformers and… | by Maxime | Inside Machine learning | Medium
- [1706.03762] Attention Is All You Need (arxiv.org)
- ChatGPT: Optimizing Language Models for Dialogue (openai.com)
Senior Data Scientist at Big Data Institute (Public Organization), BDI
- Amorn Chokchaisiripakdeehttps://temp.bdi.or.th/en/author/amorn-ch/
- Amorn Chokchaisiripakdeehttps://temp.bdi.or.th/en/author/amorn-ch/25 December 2023
Senior Developer at Big Data Institute (Public Organization), BDI
- Ananwat Tippawathttps://temp.bdi.or.th/en/author/ananwat-ti/
- Ananwat Tippawathttps://temp.bdi.or.th/en/author/ananwat-ti/
- Ananwat Tippawathttps://temp.bdi.or.th/en/author/ananwat-ti/
- Ananwat Tippawathttps://temp.bdi.or.th/en/author/ananwat-ti/