สวัสดีครับคุณผู้อ่าน วันนี้ผมจะมาแนะนำการทำโมเดล recommendation อย่างง่าย ๆ ด้วย library ที่ชื่อว่า LightFM กันนะครับ
ก่อนอื่นเลยเรามาทำความรู้จักกับเจ้า library ตัวนี้กันสักนิดนะครับ LightFM เป็นโมเดล recommendation แบบ hybrid ซึ่งถูกพัฒนาโดยบริษัทสินค้าแฟชั่นชื่อดังอย่าง lyst ที่สามารถแนะนำได้ทั้งแบบ content-based filtering และ collaborative filtering โดยผมจะขอยกตัวอย่างประกอบง่าย ๆ ของการแนะนำในแต่ละแบบนะครับ
content-based filtering นั้นเป็นการแนะนำตามคุณสมบัติของสิ่งที่เราจะแนะนำครับ เช่น ถ้าโมเดลของเรารู้ว่าเราชอบกินต้มยำไก่ และรู้ว่าต้มยำนั้นเป็นอาหารประเภทซุป ประกอบไปด้วย ไก่, ข่า, ตะไคร้, ใบมะกรูด ถ้าวันไหนเราเบื่อต้มยำ อยากกินอย่างอื่น โมเดลก็จะแนะนำให้เรากินต้มข่าไก่แทนครับ เพราะเป็นอาหารประเภทซุปเหมือนกัน และประกอบไปด้วย ไก่, ข่า, ตะไคร้, ใบมะกรูด ครับ
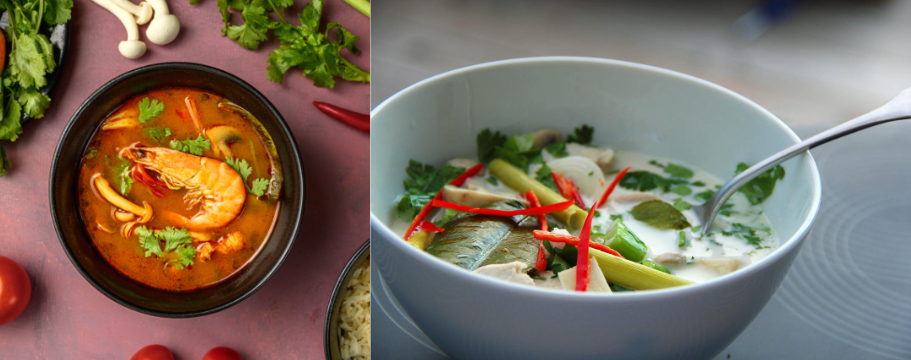
ส่วน collaborative filtering จะแนะนำสิ่งที่เราน่าจะชอบจากกลุ่มคนที่ชอบอะไรเหมือน ๆ กับเราครับ เช่น เราชอบกินข้าวหมูกรอบ และหลาย ๆ คนที่ชอบกินข้าวหมูกรอบส่วนมากก็จะกิน ผัดคะน้าหมูกกรอบ และ กระเพราหมูกรอบด้วย ดังนั้น โมเดลก็จะแนะนำให้เรากินผัดคะน้าหมูกรอบและกระเพราหมูกรอบครับ
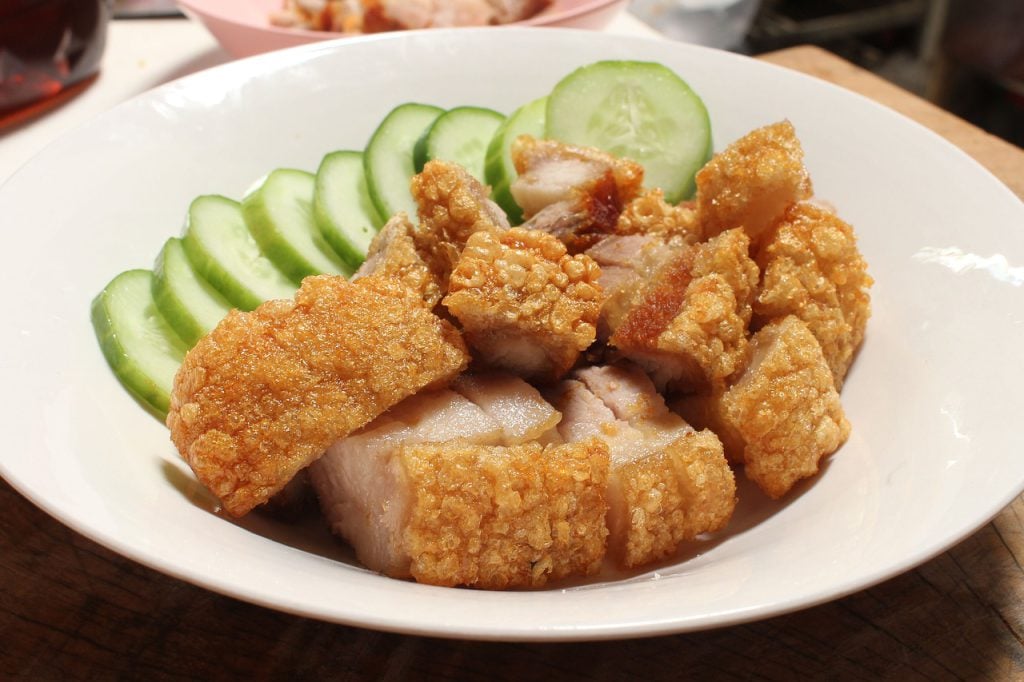
ฟังดูน่าสนใจแล้วใช่ไหมครับ งั้นเรามาลองเริ่มทำโมเดลกันเลย โดยโมเดลนี้จะเป็นการแนะนำภาพยนตร์จากชุดข้อมูล movielens ซึ่งเป็นชุดข้อมูลที่ติดมากับโมเดลอยู่แล้ว เราไม่ต้องไปหามาเพิ่มแต่อย่างใด
ขั้นตอนแรก คือการเตรียมสิ่งที่ต้องใช้ก่อนนะครับ โดยทำการติดตั้งแพ็กเกจต่าง ๆ ที่เกี่ยวข้องดังนี้ครับ
pip install lightfm numpy scipy
จากนั้นเราก็สร้างไฟล์ขึ้นมาตามแต่สะดวกจะเป็น python file หรือ python notebook เพื่อใช้สร้างโมเดลของเรา ทำการ import library กันก่อนนะครับ
import numpy as np
from lightfm.datasets import fetch_movielens
from lightfm import LightFM
ในตัวอย่างนี้เราจะใช้การแนะนำที่คำนวณจาก loss function ที่ชื่อว่า warp ซึ่งจริง ๆ มีตัวอื่น อย่าง brp, k-os warp และ logistic ให้เราเลือกด้วย โดยเงื่อนไขของข้อมูลที่เราจะ train ด้วย warp นั้น จะต้องเป็นข้อมูลภาพยนตร์ที่เขาชอบเท่านั้นครับ ดังนั้นเราจึงเลือกที่จะดึงข้อมูลคะแนน rating ภาพยนตร์มาโดยเลือกแค่ rating ที่ไม่น้อยกว่า 4
data = fetch_movielens(min_rating = 4.0)
จากนั้นเราก็ทำการสร้างโมเดล
model = LightFM(loss = ‘warp’)
และนำข้อมูลไป train ครับ โดย num_threads คือ จำนวนแกนประมวลผลในเครื่องที่เราจะใช้ในการคำนวณโมเดลครับ ในที่นี้ใส่เบื้องต้นไว้เป็น 2
model.fit(data[‘train’], epochs=30, num_threads=2)
เพียงเท่านี้เราก็จะได้โมเดลมาแล้วครับ หลังจากนี้จะเป็นการนำโมเดลมาใช้แนะนำภาพยนตร์ที่เราชอบ เราจะสร้างฟังก์ชันขึ้นมา ช่วยในการแนะนำครับ
def sample_recommendation(model, data, user_ids):
#หาจำนวนผู้ใช้และจำนวนหนังในข้อมูล
n_users, n_items = data['train'].shape
for user_id in user_ids:
#หาหนังที่ผู้ใช้ชอบอยู่แล้ว
known_positives = data['item_labels'][data['train'].tocsr()[user_id].indices]
#หาหนังที่ผู้ใช้น่าจะชอบ
scores = model.predict(user_id, np.arange(n_items))
#เรียงหนังที่จะแนะนำด้วยคะแนนของการแนะนำ
top_items = data['item_labels'][np.argsort(-scores)]
#แสดงผลลัพธ์
print("User %s" % user_id)
print(" Known positives:")
for x in known_positives[:3]:
print(" %s" % x)
print(" Recommended:")
for x in top_items[:3]:
print(" %s" % x)
เมื่อเราให้โมเดลแนะนำภาพยนตร์สำหรับ user 3, 25, 451 จะเรียกใช้ฟังก์ชันได้แบบนี้
sample_recommendation(model, data, [3, 25, 451])
และนี่ก็คือผลลัพธ์ที่ได้
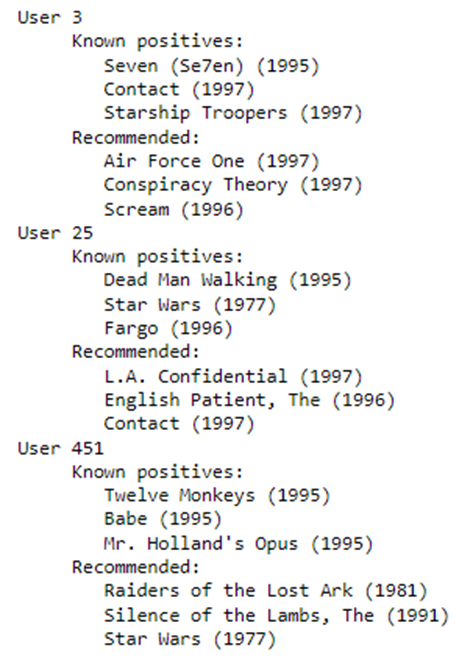
โดยสรุปแล้วการแนะนำผ่าน library lightFM นั้นสามารถทำได้ง่าย ๆ เพียงไม่กี่บรรทัดเท่านั้น โดยผลการแนะนำอาจมีความแตกต่างกันไปเพราะมีการสุ่มตัวอย่างในการ train โมเดลครับ สำหรับท่านที่อยากจะศึกษาเพิ่มเติม ผมได้แปะลิงก์อ้างอิงและลิงก์ที่น่าสนใจไว้ด้านล่างนะครับ ไว้มีโอกาสเราจะมาเจาะลึกการทำงานของ library ตัวนี้กันครับ
LightFM’s Documentation
LightFM’s Github
How to build a Movie Recommender System in Python using LightFm
Senior Data Engineer
Big Data Institute (Public Organization), BDI
- Chainarong Tumaphahttps://temp.bdi.or.th/en/author/chainarong-tu/
- Chainarong Tumaphahttps://temp.bdi.or.th/en/author/chainarong-tu/8 November 2023
- Chainarong Tumaphahttps://temp.bdi.or.th/en/author/chainarong-tu/10 October 2022
- Chainarong Tumaphahttps://temp.bdi.or.th/en/author/chainarong-tu/23 March 2021