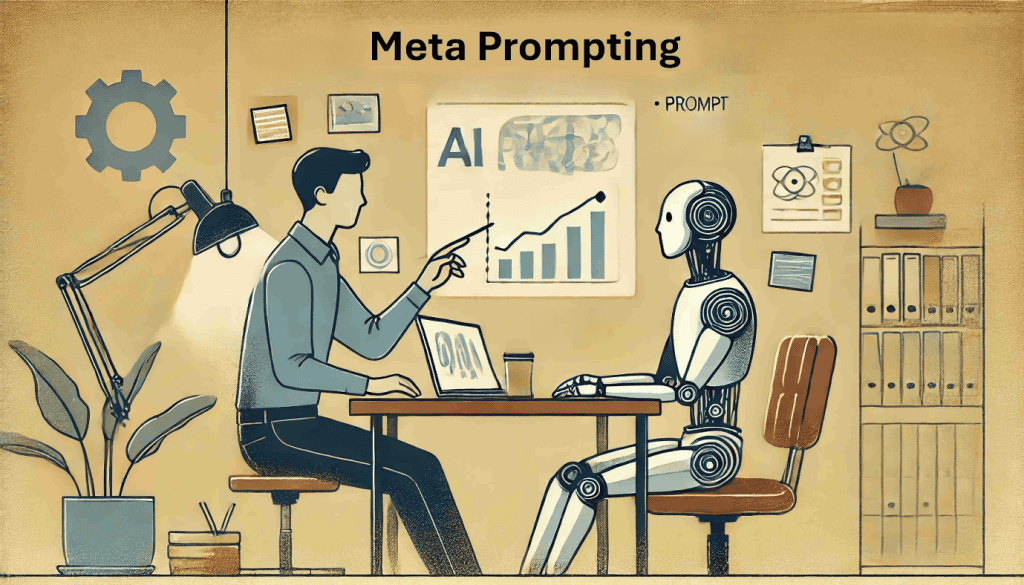
ทุกวันนี้ เราใช้ AI อย่าง ChatGPT, Claude, หรือ DeepSeek ช่วยทำงานได้หลายอย่าง ไม่ว่าจะเขียนบทความ ตอบคำถาม หรือช่วยคิดวิเคราะห์ข้อมูลต่าง ๆ แต่การจะ “สั่ง” Artificial Inteligence (AI) ให้ทำงานได้ตรงใจนั้นไม่ใช่เรื่องง่าย เพราะบางที AI ก็ให้คำตอบที่ไม่ตรงตามความต้องการ ทำให้เราต้องเสียเวลาแก้คำสั่งซ้ำไปซ้ำมา ซึ่งบทความนี้ผู้เขียนจะแนะนำเทคนิค “เมตาพรอมต์ติ้ง” หรือ Meta Prompting ที่จะให้ AI มาช่วยเราสร้างและปรับปรุง “คำสั่ง” ให้ดีขึ้นไปอีก
Meta Prompting คืออะไร?
Meta Prompting เป็นเทคนิคหนึ่งในการออกแบบคำสั่ง (Prompt Engineering) โดยเราจะใช้ AI มาช่วยสร้างหรือปรับปรุงชุดคำสั่ง แทนที่เราจะต้องคิดคำสั่งเองทั้งหมด เราก็ให้ AI ช่วยคิดโครงสร้าง เนื้อหา หรือแม้แต่ปรับปรุงคำสั่งเดิม ทำให้เราทำงานที่ซับซ้อน ได้ง่ายขึ้น และปรับตัวตามสถานการณ์ต่างๆ ได้ดีขึ้นด้วย
ทำไมต้องใช้ Meta Prompting?
- ประหยัดเวลา: ไม่ต้องเริ่มคิดคำสั่งใหม่ทั้งหมด หรือลองผิดลองถูกเอง
- ได้ผลลัพธ์ดีกว่า: คำสั่งที่ผ่านการปรับปรุงจาก AI มักจะให้ผลลัพธ์ที่ดีกว่าเราคิดเองในหลายๆครั้ง
- ปรับปรุงได้เรื่อย ๆ: เราปรับปรุงคำสั่งได้ตลอดเวลา โดยดูจากผลลัพธ์ที่ได้ และใช้ข้อมูลที่มีอยู่มาพัฒนาคำสั่งให้ดียิ่งขึ้น
วิธีการของ Meta Prompting
บทความนี้จะแนะนำตัวอย่างการทำ Meta Prompting 3 วิธี ดังนี้
1. Meta-Prompting
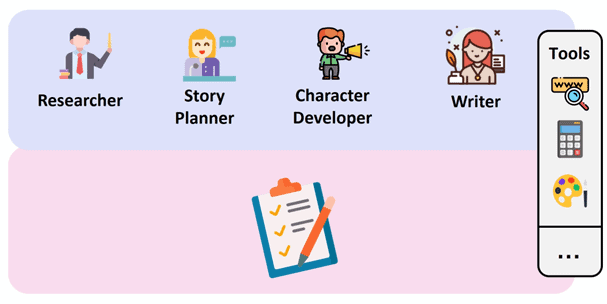
รูปที่ 1 รูปแบบการทำงานของ Meta-Prompting [1]
หลักการการทำงาน คือ การสร้าง “คำสั่งหลัก” ที่ทำหน้าที่เหมือน “ผู้จัดการโครงการ” โดยคอยแบ่งงานย่อย ๆ ให้ “คำสั่งย่อย” ที่ทำหน้าที่เหมือน “ผู้เชี่ยวชาญ” ในแต่ละด้าน เช่น “ผู้เชี่ยวชาญด้านการตลาด”, “ผู้เชี่ยวชาญด้านการเขียนโปรแกรม” แล้วค่อยรวบรวมผลลัพธ์ สามารถอ่านรายละเอียดเพิ่มเติมได้ที่ https://arxiv.org/pdf/2401.12954
ขั้นตอนการทำงาน:
- สร้าง “ผู้จัดการโครงการ” (Meta-Expert) โดยให้ “คำสั่งหลัก” (Meta Prompt) บอกให้แบ่งงานเป็นงานย่อย ๆ
- ส่งงานย่อยแต่ละงานให้ “ผู้เชี่ยวชาญ” แต่ละคน พร้อมคำสั่งที่เฉพาะเจาะจง
- ให้ “ผู้จัดการโครงการ” ประสานงาน, รวบรวม, กลั่นกรอง, และสร้างผลลัพธ์สุดท้าย
ตัวอย่างการใช้งาน:
“`
คุณคือ Meta-Expert ผู้เชี่ยวชาญด้านการวางแผนงานอีเวนต์ สามารถทำงานร่วมกับผู้เชี่ยวชาญอื่น ๆ ได้ เพื่อจัดงานเลี้ยงบริษัทให้สมบูรณ์แบบ คุณสามารถเรียกใช้ผู้เชี่ยวชาญด้านต่าง ๆ ได้แก่ “เชฟมืออาชีพ”, “นักตกแต่งสถานที่”, และ “นักวางแผนกิจกรรม”
ในการเรียกผู้เชี่ยวชาญ ให้พิมพ์ชื่อตามด้วย “:” เช่น:
เชฟมืออาชีพ: แนะนำเมนูอาหารสำหรับงานเลี้ยงบริษัท 100 คน เน้นอาหารไทย มีตัวเลือกสำหรับคนทานมังสวิรัติ และคนแพ้อาหารทะเล
นักตกแต่งสถานที่: ออกแบบการตกแต่งสำหรับงานเลี้ยงบริษัทในธีม “รื่นเริงริมทะเล” ใช้งบประมาณไม่เกิน 50,000 บาท
นักวางแผนกิจกรรม: จัดกิจกรรมสันทนาการ 3 กิจกรรมสำหรับพนักงานบริษัท เน้นกิจกรรมที่ส่งเสริมความสามัคคี และสนุกสนาน
“`
2. Learning from Contrastive Prompts
หลักการการทำงาน คือ การให้ AI เปรียบเทียบ “คำสั่งที่ดี” (ที่สามารถให้ผลลัพธ์ตรงตามความต้องการ) กับ “คำสั่งที่ไม่ดี” เพื่อเรียนรู้ว่าอะไรใช้ได้ผลและอะไรใช้ไม่ได้ผล แล้วสามารถสร้างคำสั่งใหม่ที่ดีกว่าเดิม สามารถอ่านรายละเอียดเพิ่มเติมได้ที่ https://arxiv.org/pdf/2409.15199
ขั้นตอนการทำงาน:
- เตรียมคำสั่งเริ่มต้น ตัวอย่างข้อมูล คำตอบที่ถูกต้อง และสร้างคำสั่งหลายๆ แบบ
- ให้ AI สร้างผลลัพธ์จากแต่ละคำสั่ง และประเมินว่าคำสั่งไหนดีหรือไม่ดี
- เปรียบเทียบคำสั่งที่ดีและไม่ดี เพื่อดูจุดแข็งจุดอ่อน
- ให้ AI สร้างคำสั่งใหม่ที่ปรับปรุงแล้ว และทำซ้ำขั้นตอนเหล่านี้
รูปแบบการใช้งาน
“`
โจทย์: {{ Question }}
กำหนดอินพุต: {{ Input }}
และเอาต์พุตที่คาดหวัง: {{ Output }}
อธิบายเหตุผลว่าเหตุใดอินพุตจึงสอดคล้องกับเอาต์พุตที่คาดหวัง จากนั้นใช้เหตุผลดังกล่าวสร้างพรอมต์เพื่อให้ได้เอาต์พุตที่คาดหวังจากอินพุตใดๆ ทั้งหมด 5 พรอมต์ ทำการอธิบายว่าพรอมต์ใดทำงานตามโจทย์ได้ดีที่สุด และสรุปเป็นพรอมต์ใหม่ ที่ดีขึ้นกว่าเดิม
“`
ตัวอย่างการใช้งาน
“`
โจทย์: จำแนกความรู้สึกของข้อความต่อไปนี้ว่าเป็น “บวก” หรือ “ลบ”
ข้อมูล: “หนังเรื่องนี้สนุกมาก!“
คำตอบที่ถูกต้อง: บวก
ข้อมูล: “ฉันไม่ชอบอาหารร้านนี้เลย”
คำตอบที่ถูกต้อง: ลบ
อธิบายเหตุผลว่าเหตุใดอินพุตจึงสอดคล้องกับเอาต์พุตที่คาดหวัง จากนั้นใช้เหตุผลดังกล่าวสร้างพรอมต์เพื่อให้ได้เอาต์พุตที่คาดหวังจากอินพุตใดๆ ทั้งหมด 5 พรอมต์ ทำการอธิบายว่าพรอมต์ใดทำงานตาม โจทย์ได้ดีที่สุด และสรุปเป็นพรอมต์ใหม่ ที่ดีขึ้นกว่าเดิม
“`
3. Declarative Self-improving Python (DSPy)
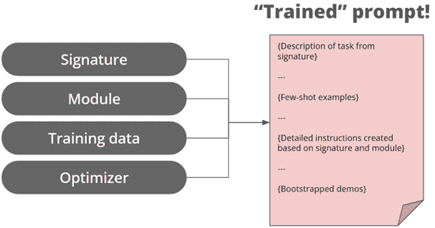
รูปที่ 2 ตัวอย่างการทำงานขอ DSPy [6]
หลักการการทำงาน: เป็นหนึ่งใน Python Library ที่มี Framework ที่ช่วยในการคอยปรับแต่งและพัฒนาคำสั่งให้ดีขึ้นโดยอัตโนมัติ สามารถอ่านรายละเอียดเพิ่มเติมได้ที่ https://arxiv.org/pdf/2310.03714
ขั้นตอนการทำงาน:
- ผู้ใช้กำหนด “โมดูล” (Modules) สำหรับงานต่างๆ (เช่น การแยกประเภทข้อความ) แต่ละโมดูลจะมี “ลายเซ็น” (Signature) บอกว่าต้องการข้อมูลเข้า (Input) แบบไหน และจะให้ข้อมูลออก (Output) แบบไหน
- DSPy จะสร้างลำดับการเรียกใช้ AI
- DSPy ปรับปรุงผลลัพธ์ไปเรื่อยๆ โดยใช้โมดูล เช่น ChainOfThought (ช่วยให้ AI คิดอย่างเป็นเหตุเป็นผล)
- โมดูล Optimizer จะประเมินว่า AI ทำงานได้ดีแค่ไหน, ปรับปรุงคำสั่ง, และปรับปรุงคุณภาพโดยรวม
ตัวอย่างการใช้งาน
“`
import dspy
# กำหนด LLM ที่จะใช้ (ในตัวอย่างนี้ขอสมมติเป็น OpenAI)
lm = lm = dspy.LM(‘openai/gpt-4o-mini’, api_key=’YOUR_OPENAI_API_KEY’)
dspy.settings.configure(lm=lm)
# กำหนด signature บอกว่า input คืออะไร (text) และ output คืออะไร (sentiment)
class SentimentAnalysis(dspy.Signature):
text = dspy.InputField(desc=”The text to analyze”)
sentiment = dspy.OutputField(desc=”Either ‘positive’ or ‘negative'”)
# สร้าง module ที่ใช้ signature นี้ และมี dspy.Predict เพื่อเรียกใช้ LLM
class AnalyzeSentiment(dspy.Module):
def __init__(self):
super().__init__()
self.classify = dspy.Predict(SentimentAnalysis)
def forward(self, text):
pred = self.classify(text=text)
return pred
# สร้างตัวอย่างข้อมูล (input, output)
trainset = [ dspy.Example(text=”หนังเรื่องนี้สนุกมาก!”, sentiment=”positive”).with_inputs(“text”), dspy.Example(text=”ฉันไม่ชอบอาหารร้านนี้เลย”, sentiment=”negative”).with_inputs(“text”)]
# เลือกวิธีปรับปรุง (ตัวอย่างนี้คือ BootstrapFewShot) และกำหนด metric (วิธีวัดความถูกต้อง)
optimizer = dspy.BootstrapFewShot(metric=lambda example, pred, trace: pred.sentiment == example.sentiment)
compiled_sentiment_analyzer = optimizer.compile(AnalyzeSentiment(), trainset=trainset)
# ทดสอบใช้งาน
result = compiled_sentiment_analyzer(text=”ร้านนี้บริการดีมาก”)
print(result.sentiment)
“`
ตารางแสดงการเปรียบเทียบของ 3 วิธี
วิธีการ | จุดเด่น | จุดด้อย | เหมาะกับ |
Meta-Prompting | จำลองผู้เชี่ยวชาญให้มาทำงานร่วมกัน | ต้องการการออกแบบคำสั่งที่มีโครงสร้างชัดเจนและอาจซับซ้อน | งานที่ต้องการความรู้หลายด้าน |
Learning from Contrastive Prompts | ปรับปรุงประสิทธิภาพของ AI โดยให้เรียนรู้จากตัวอย่างคำสั่งที่ดีและไม่ดี | ต้องการชุดข้อมูลที่มีตัวอย่างคำสั่งที่หลากหลายเพื่อการฝึกฝน | งานทั่วไป ที่ต้องการปรับปรุงคำสั่ง ให้ AI เก่งขึ้น |
DSPy | Framework ที่ช่วยในการพัฒนาและปรับปรุงระบบ AI อย่างเป็นระบบ | ต้องการความรู้ด้านการเขียนโปรแกรม Python และเวลาในการเรียนรู้การใช้งานเครื่องมือ | งานที่ซับซ้อนและต้องการโครงสร้างที่ยืดหยุ่นเมื่อพบกับชุดข้อมูลใหม่ |
สรุป
ในบทความนี้ เราได้สำรวจเทคนิค Meta Prompting ซึ่งใช้ AI เพื่อสร้างและปรับปรุงคำสั่งที่ใช้สั่งงาน AI เอง เพื่อให้ได้ผลลัพธ์ที่มีประสิทธิภาพและประหยัดเวลา เราได้พิจารณา 3 วิธีหลัก ได้แก่ Meta-Prompting ที่ใช้โครงสร้างและไวยากรณ์เพื่อให้ AI เข้าใจและสร้างคำตอบที่ซับซ้อนขึ้น Learning from Contrastive Prompts ที่ให้ AI เรียนรู้จากตัวอย่างคำสั่งที่ดีและไม่ดี เพื่อปรับปรุงความสามารถในการสร้างคำสั่งที่มีประสิทธิภาพยิ่งขึ้น และ DSPy ไลบรารี Python ที่ช่วยในการพัฒนาและปรับปรุงระบบ AI อย่างเป็นระบบ โดยการสร้างขั้นตอนการทำงานที่สามารถปรับใช้กับข้อมูลใหม่ได้อย่างง่ายดาย ทั้งนี้ การเลือกใช้วิธีใดขึ้นอยู่กับลักษณะของงานและความต้องการเฉพาะของเรา
เอกสารอ้างอิง
- Zhou, M. et al. (2023). AutoAgents: A Framework for Automatic Agent Generation. arXiv. https://arxiv.org/pdf/2309.17288
- PromptHub (2025). A Complete Guide to Meta Prompting. https://www.prompthub.us/blog/a-complete-guide-to-meta-prompting
- Wang, X. et al. (2024). Meta-Prompting: Enhancing Language Models with Task-Agnostic Scaffolding. arXiv. https://arxiv.org/pdf/2401.12954
- Li, Y. et al. (2024). Learning from Contrastive Prompts: Automated Optimization and Adaptation. arXiv. https://arxiv.org/pdf/2409.15199
- Smith, A. et al. (2023). DSPy: Compiling Declarative Language Model Calls into Self-Improving Pipelines. arXiv. https://arxiv.org/pdf/2310.03714
- Towne, J. (2025, February 28). Prompt Like a Data Scientist: Auto Prompt Optimization and Testing with DSPy. Towards Data Science. https://towardsdatascience.com/prompt-like-a-data-scientist-auto-prompt-optimization-and-testing-with-dspy-ff699f030cb7
Data Scientist at Big Data Institute (Public Organization), BDI
- Thanakorn Thaminkaewhttps://temp.bdi.or.th/en/author/thanakorn-th/24 October 2024
- Thanakorn Thaminkaewhttps://temp.bdi.or.th/en/author/thanakorn-th/
- Thanakorn Thaminkaewhttps://temp.bdi.or.th/en/author/thanakorn-th/4 April 2022
- Thanakorn Thaminkaewhttps://temp.bdi.or.th/en/author/thanakorn-th/