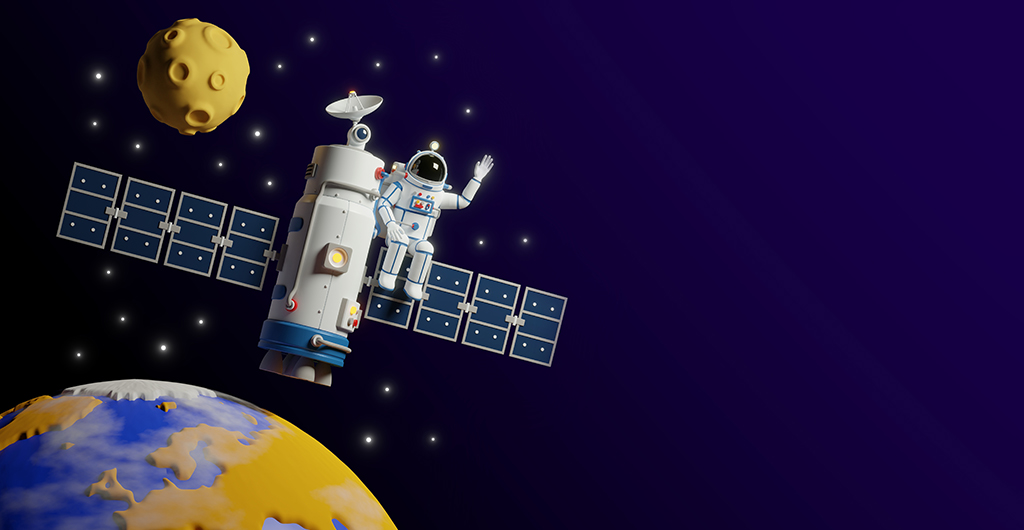
Remote Sensing หรือ การสำรวจระยะไกล เป็นกระบวนการในการตรวจจับและตรวจสอบลักษณะทางกายภาพของพื้นที่โดยการวัดรังสีที่สะท้อน และที่ปล่อยออกมาจากระยะไกล (โดยทั่วไปมาจากดาวเทียมเครื่องบิน หรือ Drone) กล้องที่มีคุณสมบัติพิเศษจะทำการถ่ายภาพจากระยะไกล ซึ่งช่วยให้เราทราบสิ่งต่าง ๆ ที่เกิดขึ้นบนโลกได้ เพราะกล้องบนดาวเทียม สามารถถ่ายภาพพื้นที่บริเวณกว้าง ทำให้มองเห็นอะไรได้มากกว่าการยืนอยู่บนพื้น
และนี่คือตัวอย่างของการนำข้อมูลภาพดาวเทียมไปใช้ในงานประยุกต์ต่าง ๆ เช่น
- ติดตามไฟป่า ข้อมูลภาพดาวเทียม ทำให้เจ้าหน้าที่ป่าไม้บริหารจัดการพื้นที่บริเวณกว้างได้
- ติดตามเมฆ เพื่อช่วยทำนายสภาพอากาศ หรือ ใช้กับระบบแจ้งเตือนเมื่อเกิดพายุ
- บริหารจัดการสถานการณ์น้ำท่วมได้อย่างมีประสิทธิภาพ
- ติดตามการเติบโตของเมือง
- ติดตามการเปลี่ยนแปลงในพื้นที่การเกษตร พื้นที่ป่าไม้ ในช่วงหลาย ๆ ทศวรรษ
- การทำแผนที่ภูมิประเทศ เช่น เทือกเขา เหว ที่ราบ มหาสมุทร
- ฯลฯ
กล้องที่มีคุณสมบัติพิเศษบนดาวเทียม จะทำให้ได้ข้อมูลที่มีคุณลักษณะแตกต่างไปจากกล้องถ่ายภาพธรรมดาที่เราใช้กันทั่วไป หากพูดถึงกล้องถ่ายภาพปกติ จะให้ข้อมูลจำนวน 3 แบนด์ (Red, Green, Blue) และ โดยปกติข้อมูลจะถูกบีบอัดและจัดเก็บในรูปแบบบิต (8-14 บิตขึ้นอยู่กับสเปคของกล้อง) ในแต่ละแบนด์ แต่หากพิจารณาข้อมูลดาวเทียม จะมีคุณสมบัติที่แตกต่างออกไป มีความพิเศษมากกว่า เนื่องจากเป็นการถ่ายภาพจากระยะไกล ทำให้ต้องเอาชนะทั้งในเรื่องของ ระยะทาง และ สิ่งรบกวนต่าง ๆ ที่อาจเกิดขึ้นในชั้นบรรยากาศ ในการเลือกข้อมูลจากดาวเทียมไปใช้งานประยุกต์ สามารถพิจารณาจากเรื่องพื้นฐานอันหนึ่ง คือ Resolutions หรือ ความละเอียด นั่นเอง สามารถแบ่งได้เป็น 4 ประเภทได้แก่ Spatial, Spectral, Radiometric และ Temporal
1. Spatial Resolution (ความละเอียดเชิงพื้นที่)
จะเป็นอันที่ เราคุ้นเคยมากที่สุด เช่น ความละเอียดเชิงพื้นที่ 10 meters (m) หมายถึง ข้อมูลนั้น แสดงวัตถุขนาด 10 x 10 sq.m. ด้วย 1 จุดภาพ (Pixel) หรือ หากเปรียบเทียบกับข้อมูลอีกชุดหนึ่งที่ความละเอียดเชิงพื้นที่ 1 m. ก็จะเป็นข้อมูลที่ละเอียดมากกว่า เมื่อเทียบกับ 10 m.
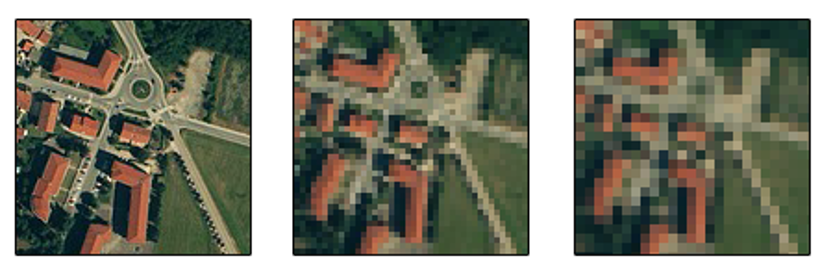
ตัวอย่าง ข้อมูลที่มีความละเอียดเชิงพื้นที่สูง (50 cm) นำมาใช้ในงานเกี่ยวกับด้านความมั่นคง ที่ต้องการเห็นรายละเอียดของวัตถุ เช่น เครื่องบินรบ รถยนต์ของผู้ก่อการร้าย
2. Spectral Resolution (ความละเอียดเชิงสเปคตรัม)
คือ ช่วงความยาวคลื่นที่ข้อมูลแต่ละชุดสามารถบ่งบอกได้ หรือ จำนวนแบนด์ของข้อมูลนั่นเอง เช่น มีแบนด์ NIR, Red, Green, Blue เรียกว่า Multi-Spectral หรือ มีแบนด์เป็นจำนวนหลักร้อย เรียกว่า Hyper-Spectral

เหตุผลที่กล้องบนดาวเทียมมีจำนวนแบนด์มากกว่ากล้องถ่ายภาพปกติ เนื่องจากระยะทางที่ไกล และ หากต้องการตรวจจับวัตถุบนพื้นโลก เช่น พื้นที่เกษตรกรรม กับ มหาสมุทร การมีหลายแบนด์ทำให้สามารถสร้าง Signature ของวัตถุต่าง ๆ ได้มีประสิทธิภาพมากกว่า
3. Radiometric Resolution (ความละเอียดเชิงรังสี)
คือ ความละเอียดของข้อมูลดาวเทียม ที่แบ่งระดับการแยกแยะความเข้ม ในแต่ละแบนด์ ตัวอย่างของรูปภาพจากกล้องปกติ คือ ข้อมูล 8 บิต แสดงค่าที่แตกต่างได้ 2^8 = 256 ระดับ สำหรับข้อมูลดาวเทียม เช่น Landsat 8 อาจมีข้อมูลแบบ 16 บิต แสดงค่าได้ 2^16 = 65,536 ระดับ ในกรณีนี้ จำนวนบิตที่มาก จะแยกแยะความเข้มได้ละเอียดมากกว่านั่นเอง
4. Temporal Resolution (ความละเอียดเชิงเวลา)
ข้อมูลภาพดาวเทียม จะถูกถ่าย ณ ช่วงเวลาหนึ่ง ค่า Temporal Resolution จะเป็นการวัดช่วงเวลาที่จะได้ข้อมูลพื้นที่บริเวณเดิมอีกครั้ง บางครั้ง เรียกว่า “Return time” หรือ “Revisit time” เป็นเวลาที่ Satellite นั้น สามารถโคจรกลับมาถ่ายภาพ ณ บริเวณเดิมได้
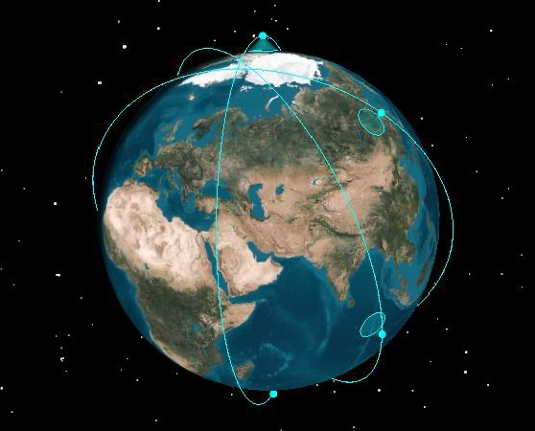
พิจารณาข้อมูล Satellite อันหนึ่ง ซึ่งมีข้อมูลทุก 16 วัน เปรียบเทียบกับข้อมูลอีกชุดหนึ่ง มีข้อมูลทุก ๆ วัน ข้อมูลชุดที่มีทุกวัน จะมีความละเอียดเชิงเวลาที่มากกว่า
หากมีความเข้าใจเรื่องพื้นฐานเหล่านี้แล้ว เราก็สามารถเลือกใช้ข้อมูล ในแต่ละงานประยุกต์ได้อย่างเหมาะสม เช่น เหตุการณ์น้ำท่วม ที่ต้องบริหารจัดการแบบรายวัน ก็เลือกข้อมูลดาวเทียมที่มีความละเอียดรายวัน การประเมินพื้นที่ป่าไม้ที่หายไป ซึ่งดูการเปลี่ยนแปลงในรอบหลาย ๆ สิบปี ก็ไม่ต้องการความละเอียดเชิงเวลา และ พื้นที่ สูงมากนัก เป็นต้น
จะเห็นได้ว่าในแง่ของข้อมูล ถือได้ว่าเป็น (Geospatial) Big Data อันหนึ่ง ในปัจจุบันมีงานวิจัยเกี่ยวกับ Remote Sensing โดยใช้ Machine Learning หรือ Deep Learning Algorithms [3] เป็นจำนวนมาก และ มีข้อมูล Open Data ที่นิยมใช้อย่างเช่น Landsat, MODIS, Sentinel, ฯลฯ [4]
ข้อมูลอ้างอิง
- https://www.usgs.gov/faqs/what-remote-sensing-and-what-it-used#:~:text=Remote%20sensing%20is%20the%20process,sense%22%20things%20about%20the%20Earth.
- https://gisgeography.com/spatial-resolution-vs-spectral-resolution/
- Deep learning in remote sensing applications: A meta-analysis and review by Lei Ma et al. https://www.sciencedirect.com/science/article/pii/S0924271619301108
- 15 Free Satellite Imagery Data Sources by GISGeography https://gisgeography.com/free-satellite-imagery-data-list/
- หน่วยงานภาครัฐของไทย www.gistda.or.th
เนื้อหาโดย ดร.นรุตม์ สุนทรานนท์
ตรวจทานและปรับปรุงโดย นววิทย์ พงศ์อนันต์
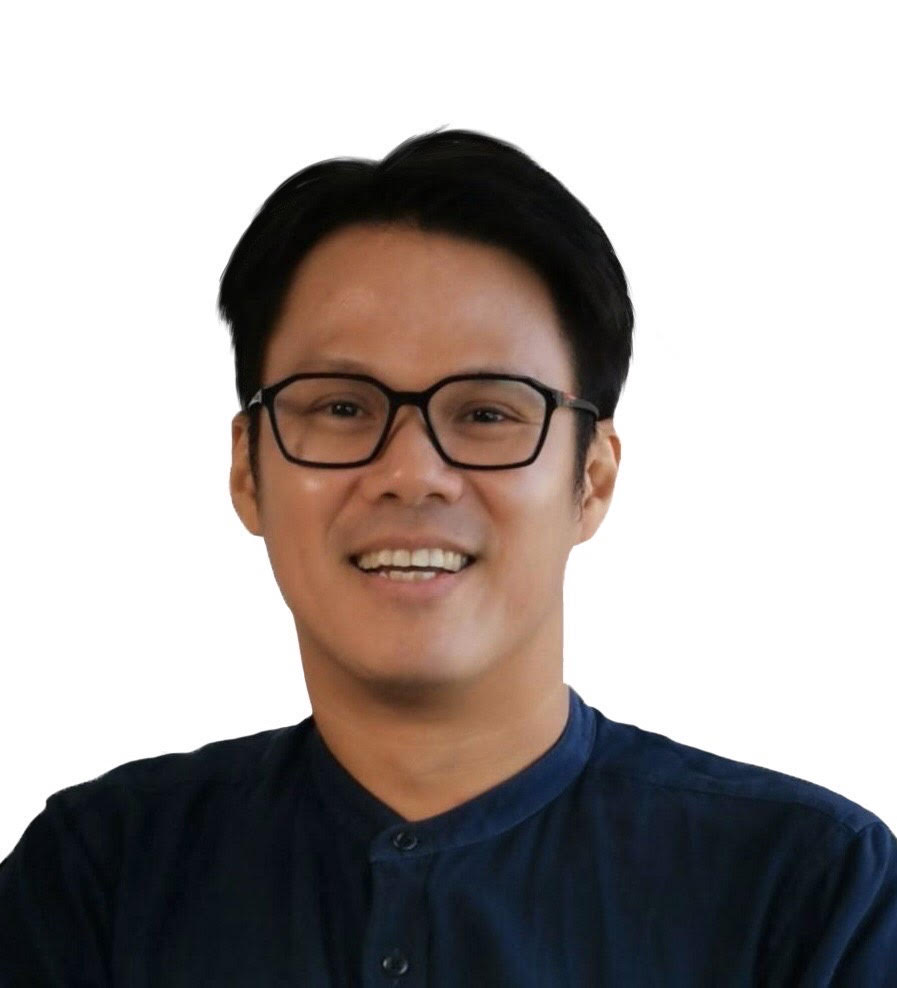
Narut Soontranon
Page's admin of Nerd (@nerd.data)
- This author does not have any more posts.
- Navavit Pongananhttps://temp.bdi.or.th/en/author/gas/30 August 2024
- Navavit Pongananhttps://temp.bdi.or.th/en/author/gas/
- Navavit Pongananhttps://temp.bdi.or.th/en/author/gas/
- Navavit Pongananhttps://temp.bdi.or.th/en/author/gas/