ในปี 2016 Deepmind ได้แสดงความสามารถของ AI AlphaGo ที่สามารถเอาชนะแชมป์โลกในเกมกระดานอย่างหมากล้อม ซึ่งเป็นเกมที่โปรแกรมทั่วไปไม่สามารถเอาชนะมนุษย์ได้มาก่อน ต่อจากนั้นมา Deepmind ก็ได้พัฒนา AI มาเรื่อย ๆ โดยมี AlphaZero และ MuZero ที่ได้ถูกพัฒนาขึ้นมาตามลำดับ การพัฒนา AI ของ Deepmind นั้นมีจุดประสงค์ที่จะทำให้ AI สามารถเรียนรู้ได้หลากหลายมากยิ่งขึ้น และใช้ความรู้เริ่มต้นน้อยลง ตัวอย่างเช่น MuZero สามารถชนะมนุษย์ในเกมหมากล้อม หมากรุก โชกิ และเกมบน Atari โดยไม่จำเป็นที่จะต้องรู้กฎของเกมเลยด้วยซ้ำ
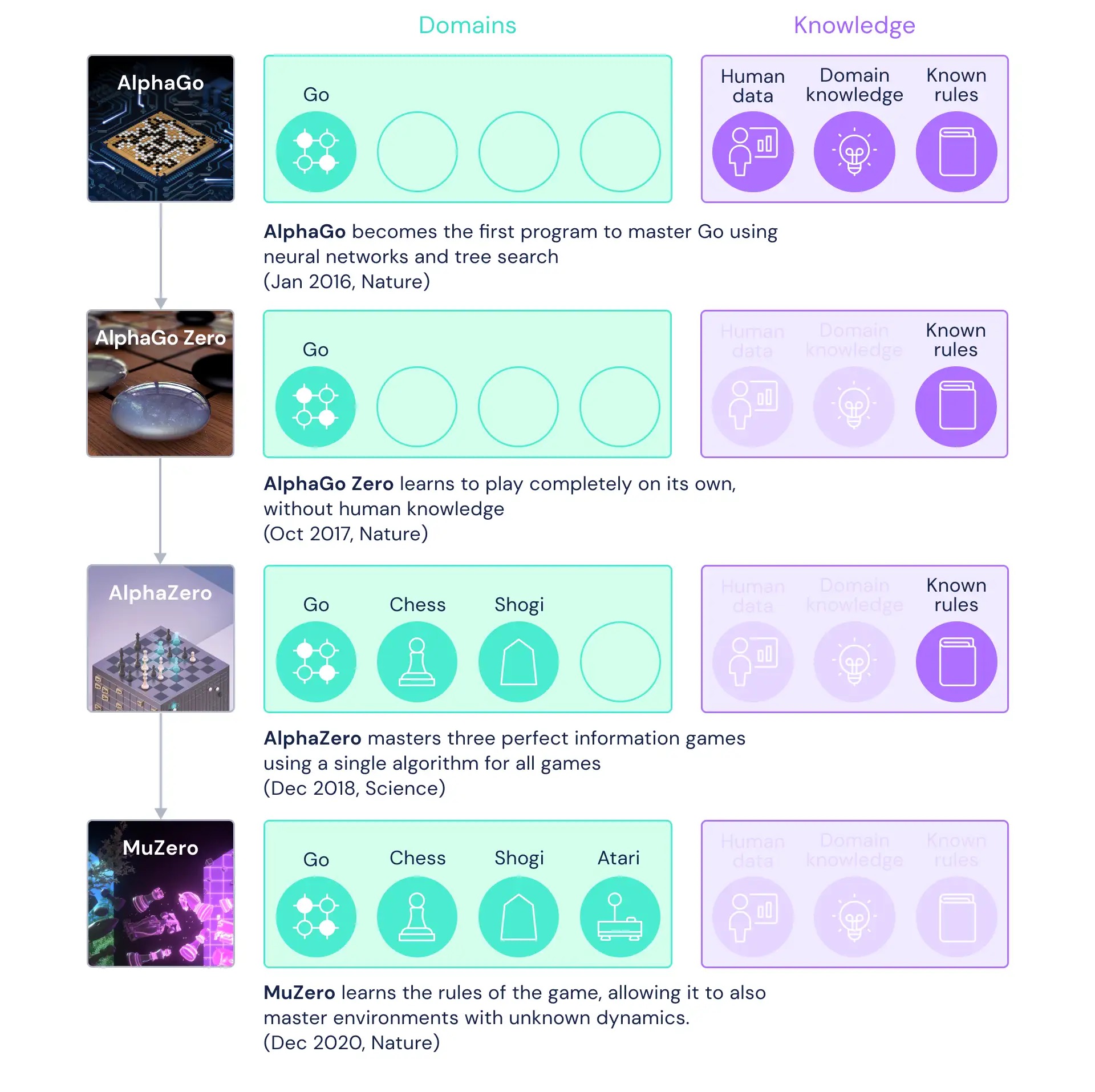
ที่มาภาพ : deepmind.com
แต่ผลงานทั้งหมดที่กล่าวมาเป็นเพียงแค่การเรียนรู้เกม Deepmind จึงได้ตั้งเป้าหมายถัดไปเป็นการแก้ไขปัญหาในชีวิตจริง โดยโจทย์แรกของ MuZero ในการก้าวเข้าสู่โลกแห่งความจริง คือการ Optimize วิดีโอบน YouTube
การ Optimize วิดีโอมีความสำคัญเป็นอย่างมาก เนื่องจากปริมาณการใช้งานวิดีโอในปัจจุบันสูงขึ้นมาก ถ้าหากทำการบีบอัดวิดีโอ (Video compression) ได้ดี ก็จะสามารถลด traffic บนอินเตอร์เน็ตได้มหาศาล และเป็นโจทย์ที่เหมาะกับ MuZero ซึ่งใช้การเรียนรู้แบบเสริมกำลัง (Reinforcement Learning: RL) เพื่อพัฒนาวิธีการย่อขนาดวิดีโอให้ดีกว่าเดิม ผลการศึกษาพบว่า MuZero สามารถลด bitrate ของวิดีโอได้เฉลี่ย 4% หากใครสนใจผลการวิจัยฉบับเต็มสามารถดูได้ที่นี่
วิดีโอในแพลตฟอร์มออนไลน์ส่วนใหญ่จะใช้โปรแกรมที่เรียกว่า codec เพื่อบีบอัดหรือเข้ารหัสวิดีโอจากต้นทางของผู้อัปโหลด ส่งข้อมูลผ่านอินเตอร์เน็ต และแปลงข้อมูลกลับเพื่อแสดงวิดีโอที่ปลายทาง โปรแกรม codec จะต้องตัดสินใจเลือกตัวเลือกหลายอย่างในทุก ๆ เฟรมของวิดีโอ โดยวิธีการตัดสินใจของ codec ก็เป็นศาสตร์ที่ได้มีการพัฒนาโดยนักวิจัยและวิศวกรมานับสิบปี อย่างไรก็ตาม อัลกอริทึมแบบ RL มีความเหมาะสมกับการแก้ปัญหาที่เกี่ยวกับการตัดสินใจอย่างต่อเนื่องแบบนี้ ทาง Deepmind จึงเห็นว่าเป็นโจทย์ที่น่าลองนำ MuZero มาเรียนรู้ และศึกษาว่าจะนำ AI มาช่วยวงการวิดีโอได้อย่างไรบ้าง
ในบทความนี้เราจะโฟกัสไปที่ VP9 codec (โดยใช้ opensource libvpx) ซึ่งเป็น codec ที่ใช้กันอย่างแพร่หลายบน YouTube ในการใช้ VP9 ผู้ใช้งานจะต้องคำนึงถึง bitrate (จำนวน 0 หรือ 1 ที่ใช้ในแต่ละเฟรมของวิดีโอ) ซึ่งค่า bitrate จะส่งผลต่อขนาดของวิดีโอเป็นอย่างมาก การทำ Optimization ใน VP9 จะทำบน Rate Control Module โดยใช้ตัวแปร Quantisation Parameter (QP) ในการปรับค่า bitrate (ค่า QP มาก จะทำให้ bitrate ต่ำ และค่า QP น้อยทำให้ bitrate สูง)
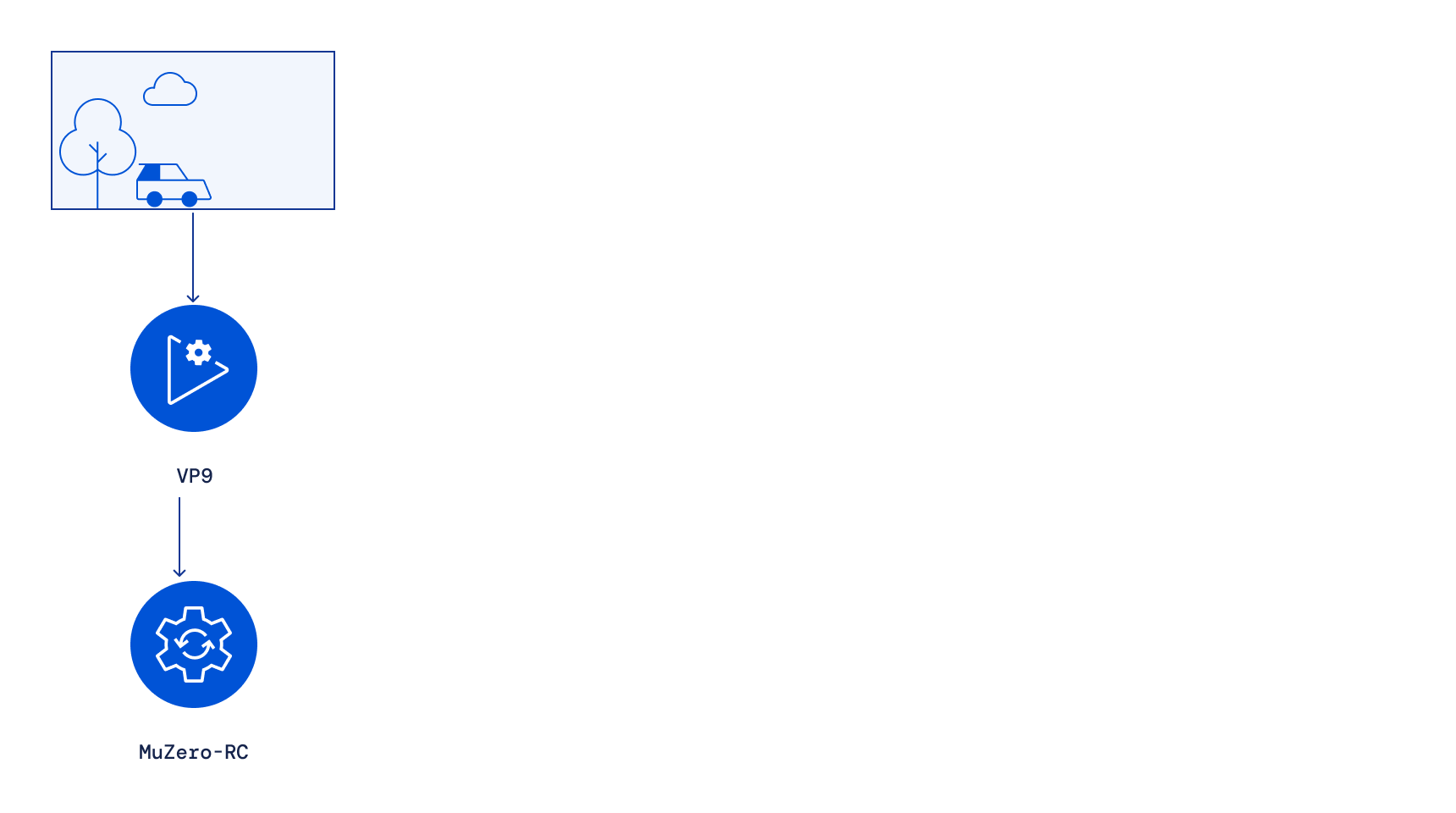
สิ่งที่เราต้องการคือ maximize คุณภาพของวิดีโอ (วัดคุณภาพจากค่า Peak Signal-to-Noise Ratio: PSNR) โดยที่มีข้อจำกัดคือขนาดของวิดีโอต้องเล็กกว่าค่าที่ผู้ใช้งานกำหนด สิ่งที่โปรแกรมจะต้องทำก็คือปรับเฟรมที่มีการเคลื่อนไหวมาก ๆ ให้มี bitrate สูง (QP ต่ำ) และในทางตรงข้าม ปรับให้เฟรมที่มีการเคลื่อนไหวน้อย ๆ ให้มี bitrate ต่ำ (QP สูง)
ปัญหาที่ Deepmind พบจากการลองให้ MuZero แก้โจทย์ Optimization ดังกล่าวโดยตรงคือ ผลที่ได้นั้นอ่อนไหวต่อค่า learning rate ของโมเดลเป็นอย่างมาก ทำให้ปรับจูนโมเดลได้ยาก นอกจากนั้น MuZero ไม่สามารถหาวิธีการที่ดีที่สุดออกมาวิธีเดียวได้ เนื่องจากวิดีโอมีความหลากหลายมาก ทาง Deepmind จึงเสนอวิธีการเรียนรู้ของโมเดลด้วยการแข่งขันกับตัวเอง โดยจะให้รางวัลโมเดลก็ต่อเมื่อโมเดลสร้างวิดีโอผลลัพธ์ที่คุณภาพดีกว่าผลงานของตัวเองในอดีต การกำหนดเป้าหมายแบบนี้เปลี่ยนการ maximize คุณภาพของวิดีโอ ให้กลายเป็นสัญญาณง่าย ๆ เพียงแค่ ชนะ หรือแพ้เท่านั้น ทำให้เทรนโมเดลได้ง่ายขึ้นมาก
ประหยัด bitrate ไปได้
4.7 %
ประหยัด bitrate ไปได้
4.1 %
ประหยัด bitrate ไปได้
3.5 %
ผลที่ได้จากการให้ MuZero เรียนรู้วิธีการ Optimize วิดีโอด้วยการแข่งขันกับตัวเอง คือโมเดล MuZero Rate-Controller (MuZero-RC) ซึ่งสามารถลดขนาดของวิดีโอได้ 4% โดยเฉลี่ย โดยที่ไม่ลดคุณภาพของวิดีโอ และมีความสามารถในการ generalize โดยผ่านการทดสอบด้วยวิดีโอที่มีความหลากหลายทั้งในเชิงเนื้อหา และคุณภาพของวิดีโอ บนแพลตฟอร์ม YouTube
ผลงานครั้งนี้ของ MuZero แสดงให้เห็นถึงศักยภาพของ AI ที่สามารถเรียนรู้วิธีการ Optimize วิดีโอได้โดยไม่ต้องใช้ข้อมูลนอกเหนือไปจากวิดีโอที่ใช้ให้ AI เรียน โดยมีผลลัพธ์ที่ไม่แย่ไปกว่าผลจากการวิจัยด้านการ Optimize codec นับสิบปี และนี่ก็คือก้าวแรกของ MuZero ในการแก้ไขปัญหาในชีวิตจริง และ Deepmind จะมุ่งหน้าสู่การสร้างอัลกอริทึมที่สามารถ แก้โจทย์ได้นับพันโจทย์ได้ด้วยอัลกอริทึมเดียว
บทความโดย MuZero Applied Team
เนื้อหาจากบทความ MuZero’s first step from research into the real world
แปลและเรียบเรียงโดย พชร วงศ์สุทธิโกศล
ตรวจทานและปรับปรุงโดย อิสระพงศ์ เอกสินชล
Associate, Research and Innovations Division (RIN)
Big Data Institute (BDI)
- Patchara Wongsutthikosonhttps://temp.bdi.or.th/en/author/patchara-wo/18 May 2023
- Patchara Wongsutthikosonhttps://temp.bdi.or.th/en/author/patchara-wo/
- Patchara Wongsutthikosonhttps://temp.bdi.or.th/en/author/patchara-wo/14 June 2021
- Patchara Wongsutthikosonhttps://temp.bdi.or.th/en/author/patchara-wo/15 February 2021
Senior Project Manager & Data Scientist at Big Data Institute (Public Organization), BDI
- Isarapong Eksinchol, PhDhttps://temp.bdi.or.th/en/author/isarapong/
- Isarapong Eksinchol, PhDhttps://temp.bdi.or.th/en/author/isarapong/
- Isarapong Eksinchol, PhDhttps://temp.bdi.or.th/en/author/isarapong/
- Isarapong Eksinchol, PhDhttps://temp.bdi.or.th/en/author/isarapong/